Google’s latest Go victory shows machines are no longer just learning, they’re teaching
Google's AlphaGo victory over the human world champion shows how far things have come since Deep Blue
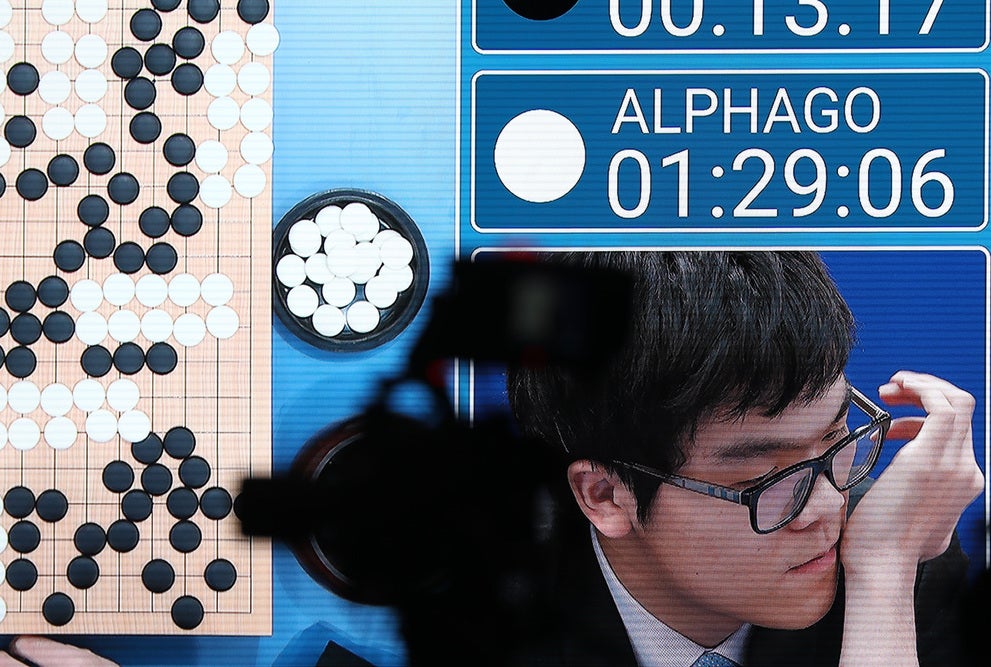
Your support helps us to tell the story
From reproductive rights to climate change to Big Tech, The Independent is on the ground when the story is developing. Whether it's investigating the financials of Elon Musk's pro-Trump PAC or producing our latest documentary, 'The A Word', which shines a light on the American women fighting for reproductive rights, we know how important it is to parse out the facts from the messaging.
At such a critical moment in US history, we need reporters on the ground. Your donation allows us to keep sending journalists to speak to both sides of the story.
The Independent is trusted by Americans across the entire political spectrum. And unlike many other quality news outlets, we choose not to lock Americans out of our reporting and analysis with paywalls. We believe quality journalism should be available to everyone, paid for by those who can afford it.
Your support makes all the difference.Just over 20 years ago a computer beat a human world champion in a chess match for the first time, when IBM’s Deep Blue supercomputer beat Garry Kasparov in a narrow victory of 3½ games to 2½. Just under a decade later, machines were deemed to have conquered the game of chess when Deep Fritz, a piece of software running on a desktop PC, beat 2006 world champion Vladimir Kramnik.
Now the ability of computers to take on humanity has taken a step further by mastering the far more complex board game Go, with Google’s AlphaGo program beating world number one Ke Jie twice in a best-of-three series.
This significant milestone shows just how far computers have come in the past 20 years. Deep Blue’s victory at chess showed machines could rapidly process huge amounts of information, paving the way for the big data revolution we see today. But AlphaGo’s triumph represents the development of real artificial intelligence by a machine that can recognise patterns and learn the best way to respond to them. What’s more, it may signify a new evolution in AI, where computers not only learn how to beat us but can start to teach us as well.
Go is considered one of the world’s most complex board games. Like chess, it’s a game of strategy but it also has several key differences that make it much harder for a computer to play. The rules are relatively simple but the strategies involved to play the game are highly complex. It is also much harder to calculate the end position and winner in the game of Go.
It has a larger board (a 19x19 grid rather than an 8x8 one) and an unlimited number of pieces, so there are many more ways that the board can be arranged. Whereas chess pieces start in set positions and can each make a limited number of moves each turn, Go starts with a blank board and players can place a piece in any of the 361 free spaces. Each game takes on average twice as many turns as chess and there are six times as many legal move options per turn.
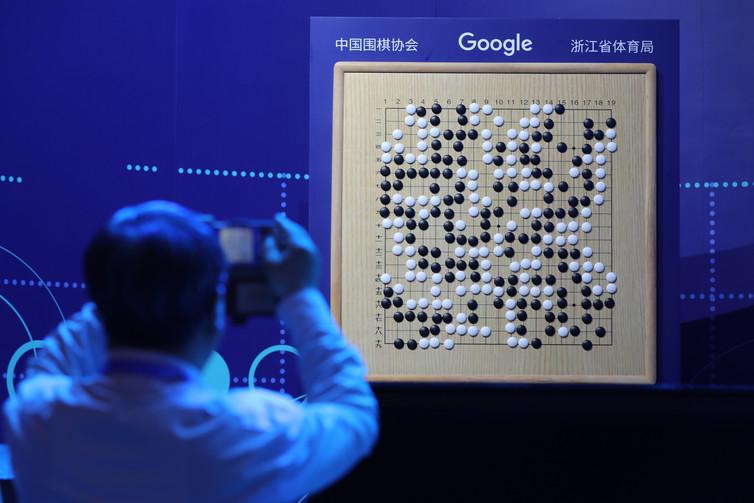
Each of these features means you can’t build a Go program using the same techniques as for chess machines. These tend to use a “brute force” approach of analysing the potential of large numbers of possible moves to select the best one. Feng-Hsiung Hsu, one of the key contributors to the DeepBlue team, argued in 2007 that applying this strategy to Go would require a million-fold increase in processing speed over DeepBlue so a computer could analyse 100 trillion positions per second.
Learning new moves
The strategy used by AlphaGo’s creators at Google subsidiary DeepMind was to create an artificial intelligence program that could learn how to identify favourable moves from useless ones. This meant it wouldn’t have to analyse all the possible moves that could be made at each turn. In preparation for its first match against professional Go player Lee Sedol, AlphaGo analysed around 300m moves made by professional Go players. It then used what are called deep learning and reinforcement learning techniques to develop its own ability to identify favourable moves.
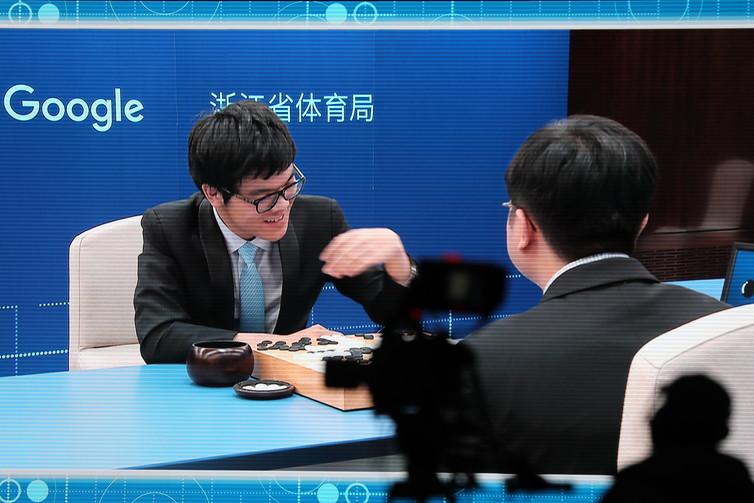
But this wasn’t enough to enable AlphaGo to defeat highly ranked human players. The software was run on custom microchips specifically designed for machine learning, known as tensor processing units (TPUs), to support very large numbers of computations. This seems similar to the approach used by the designers of DeepBlue, who also developed custom chips for high-volume computation. The stark difference, however, is that DeepBlue’s chips could only be used for playing chess. AlphaGo’s chips run Google’s general-purpose AI framework, Tensorflow, and are also used to power other Google services such as Street View and optimisation tasks in the firm’s data centres.
Lesson for us all
The other thing that has changed since Deep Blue’s victory is the respect that humans have for their computer opponents. When playing chess computers, it was common for the human players to adopt so-called anti-computer tactics. This involves making conservative moves to prevent the computer from evaluating positions effectively.
In his first match against AlphaGo, however, Ke Jie, adopted tactics that had previously been used by his opponent to beat it at its own game. Although this attempt failed, it demonstrates a change in approach for leading human players taking on computers. Instead of trying to stifle the machine, they have begun trying to learn from how it played in the past.
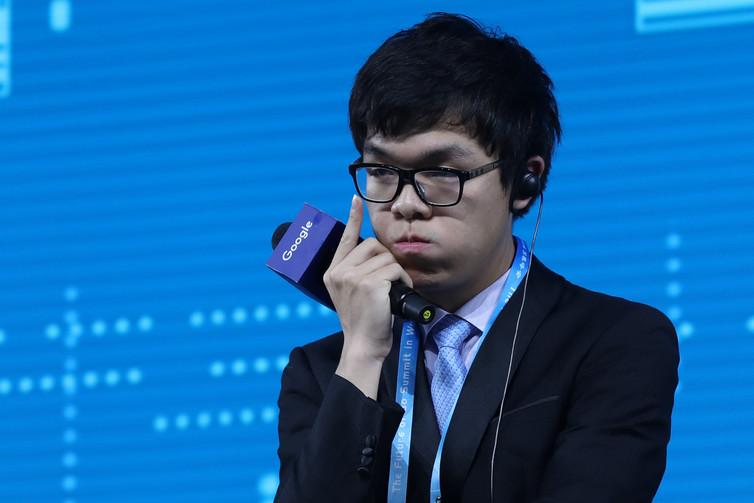
In fact, the machine has already influenced the professional game of Go, with grandmasters adopting AlphaGo’s strategy during their tournament matches. This machine has taught humanity something new about a game it has been playing for over 2,500 years, liberating us from the experience of millennia.
What then might the future hold for the AI behind AlphaGo? The success of Deep Blue triggered rapid developments that have directly impacted the techniques applied in big data processing. The benefit of the technology used to implement AlphaGo is that it can already be applied to other problems that require pattern identification.
For example, the same techniques have been applied to the detection of cancer and to create robots that can learn to do things like open doors, among many other applications. The underlying framework used in AlphaGo, Google’s TensorFlow, has been made freely available for developers and researchers to build new machine-learning programs using standard computer hardware.
More excitingly, combining it with the many computers available through the internet cloud creates the promise of delivering machine-learning supercomputing.
When this technology matures then the potential will exist for the creation of self-taught machines in wide-ranging roles that can support complex decision-making tasks. Of course, what may be even more profound are the social impacts of having machines that not only teach themselves but teach us in the process.
Mark Robert Anderson is a professor in computing and information systems at Edge Hill University. This article was originally published on The Conversation (www.conversation.com)
Join our commenting forum
Join thought-provoking conversations, follow other Independent readers and see their replies
Comments