The devil is in the dataset: Machines can be racist too
With tech’s increasing reliance on AI and algorithms, we need to ensure datasets aren’t flawed in order to reduce the danger of machines making prejudiced decisions. By Miles Ellingham
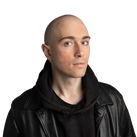
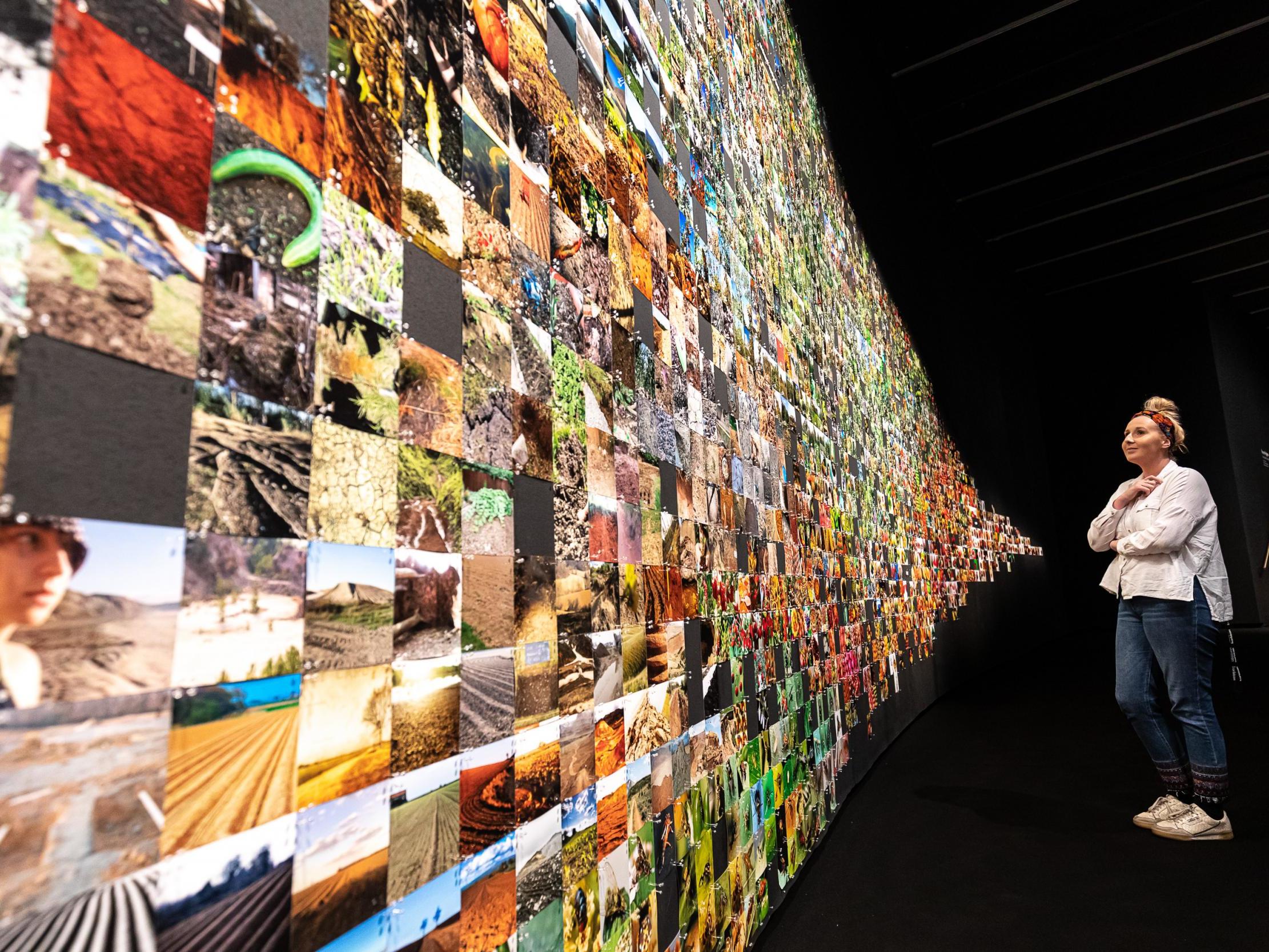
Damn racist sinks!” giggled friends TJ Fitzpatrick and Larry at the end of a YouTube video that went viral back in 2017. The sink in question belonged to Atlanta’s Marriot Hotel, which was hosting a sci-fi event when, on a toilet break, TJ and Larry realised the tap’s infrared sensor refused to dispense soap to black hands. “I tried all the soap dispensers in the restroom, there were maybe 10, and none of them worked,” TJ recalls. “Any time I went into that restroom, I had to have my friend get the soap for me…”
The two friends didn’t see this as anything too serious, just one of those odd kinks in the otherwise taut rope of technological progress. And, for the millions of people who shared the video on meme pages and message boards, TJ’s “racist sink” was an absurd joke. But without knowing it, TJ and Larry had exemplified a pernicious question at the heart of machine learning and tomorrow’s society: the question of the dataset. It is a question that may turn out to have life and death implications, and haunts everyone from programmers to artists to executives.
The dataset is a vital component in “machine learning”. That’s the process of literally teaching a programme to recognise, isolate or even invent features of our world. This encompasses a huge range of actual and potential technologies – from self-driving cars to electronic passport gates – and will become ubiquitous as technology grows ever more integrated into the minutiae of our daily lives. One technique of machine learning is an ingenious process called a GAN (a generative adversarial network), which you’ll have to understand, however darkly.
It’s like this. Have you ever used one of those apps to see what you’d look like as an old person, or as the opposite gender? Ever seen a programme that can colour a black and white photograph? A GAN makes it possible to counterfeit your geriatric features or to guess where the colour matches an otherwise colourless image.
The easiest way to understand this process is to imagine its two component parts: the generator and the discriminator – which lie at the heart of things. A GAN works by playing a sort of game between the generator (which you might think of as a forger) and the discriminator (which you might think of as a police officer, whose job it is to spot the forgery).
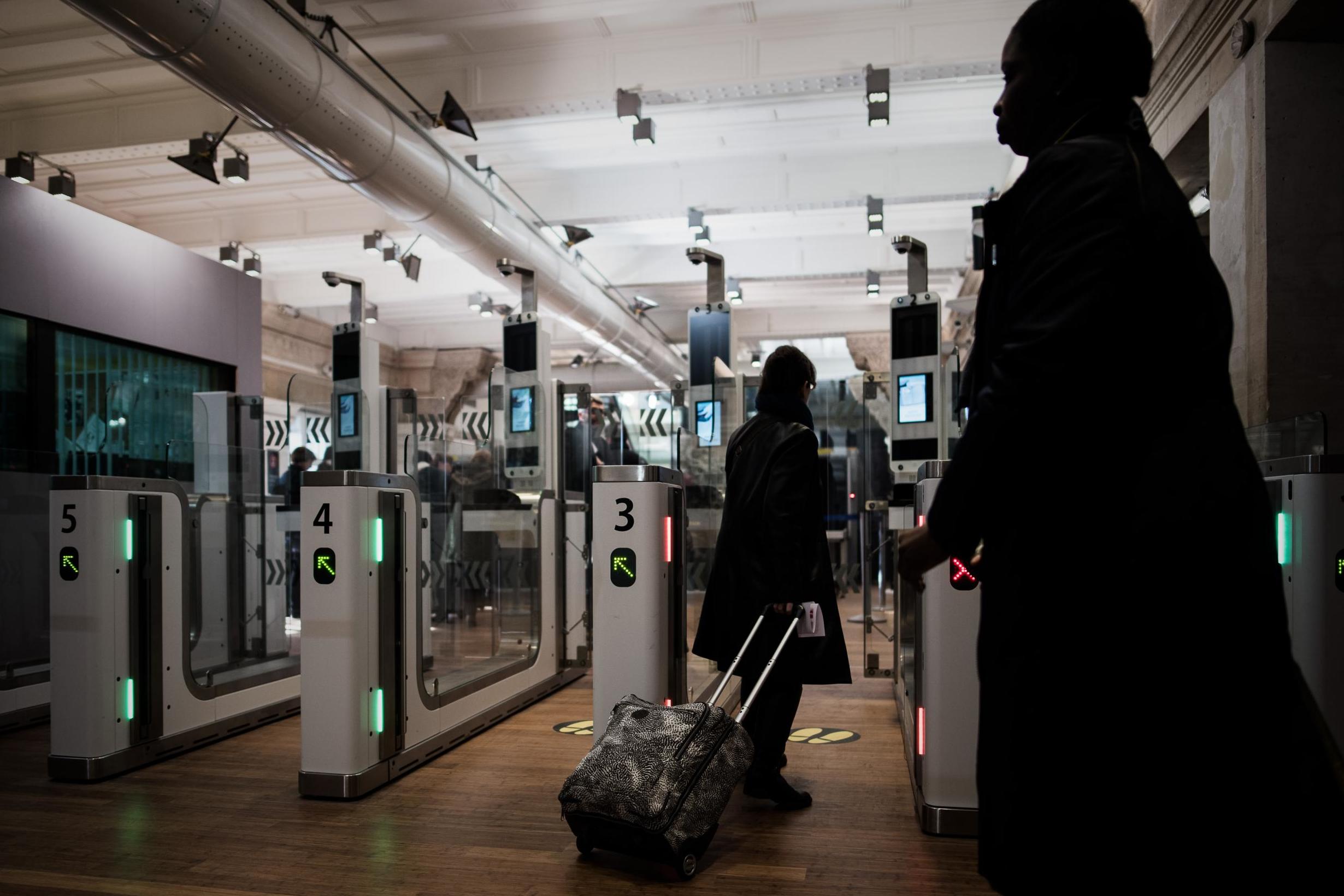
The generator’s challenge is to make an image good enough to trick the discriminator (this could be an image, like a face, but it could also be words or sounds, depending on what you train it on). It does this by reconstituting random noise into a simulation of whatever it’s trying to fake. Once it’s finished, the simulation will be put through the network and judged next to a real example of whatever that thing is – let’s say, a human face – which is taken from a dataset. The dataset is a cache of exemplary items (a huge number of genuine human faces, for instance, gathered together for easy use).
If the discriminator judges the generated image to be a forgery – which will probably be the case for the first hundred thousand iterations of the system – a message will be sent back to the generator which will fine tune accordingly. Once the generator’s fake is too realistic for the discriminator to detect, and it lets a forgery through, a message will be sent back to the discriminator, which will also fine tune itself. Through this process of adaptive conversation between generator and discriminator, eventually a hyper-realistic fake is produced. Or, indeed, a very capable discriminator, which could be used as, say, a classifier for telling an automated sink whether what’s in front of it is flesh or porcelain.
Here’s the important point, however. Based on the GAN process, what would you think is its most corruptible component? It’s the dataset. The GAN only learns to work with what it’s given and if what it’s given is biased, then the resultant network is going to be biased, too. It does exactly what it’s told.
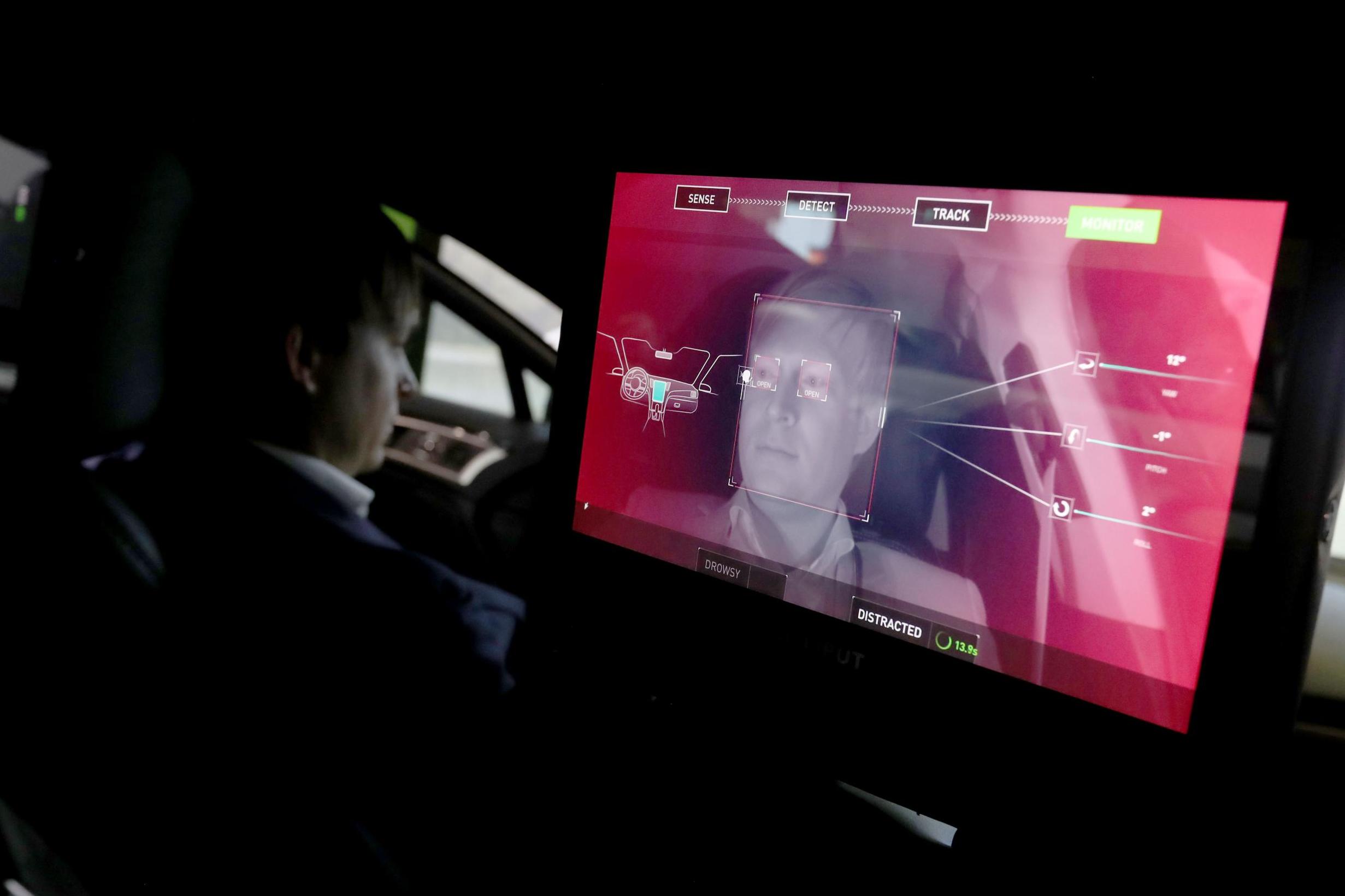
In the case of TJ’s racist sink, the problem came from a deficit of dark-skinned hands in the dataset that was employed to teach it what a hand was. Luckily for TJ, it was only a soap dispenser, and not something that could have killed or arrested him. However, what if it was a self-driving car, out of control, bearing down on him and a white friend, employing its programme to make a split-second automated decision – which body to crush? What if, because of those same biases in the dataset, such a car sees TJ as an animal?
This has, disturbingly, happened before: back in 2015, software engineer Jacky Alcine pointed out that the image recognition algorithms in Google Photos were classifying his black friends as “gorillas”. Google said it was “appalled” at the mistake but only managed to circumvent it by blocking image categories “gorilla”, “chimp”, “chimpanzee” and “monkey” entirely – the error was too deep-seated for anything less. Furthermore, in modern warfare, machine learning will play an increasingly important role as autonomous weapons are brought to the fore. What if they use flawed datasets too? What if an autonomous weapon can’t determine a woman in a burqa from an inanimate roadside object?
AI often has this mystification about it where it’s this autonomous thing. What’s much scarier is the fact that it’s a reflection of our own political views and biases
The politics of the dataset is going to be a vital conversation as technology becomes increasingly indispensable and we all turn cyborg. It’s already an active issue. In the US, black people have been wrongfully convicted due to dataset bias. The American Civil Liberties Union is currently demanding action after a man in Detroit was cuffed and thrown in jail thanks to facial recognition misidentification.
One of the key things to dispel in our modern technological story is that technology is anything other than a reflection of ourselves. The reason Hal 9000 refuses to open the pod bay doors may well lie with a particularly acrimonious programmer. Whoever put Cyberdyne Systems Model 101 aka Terminator together likely watched too many action movies themselves. Technology is as flawed as we are.
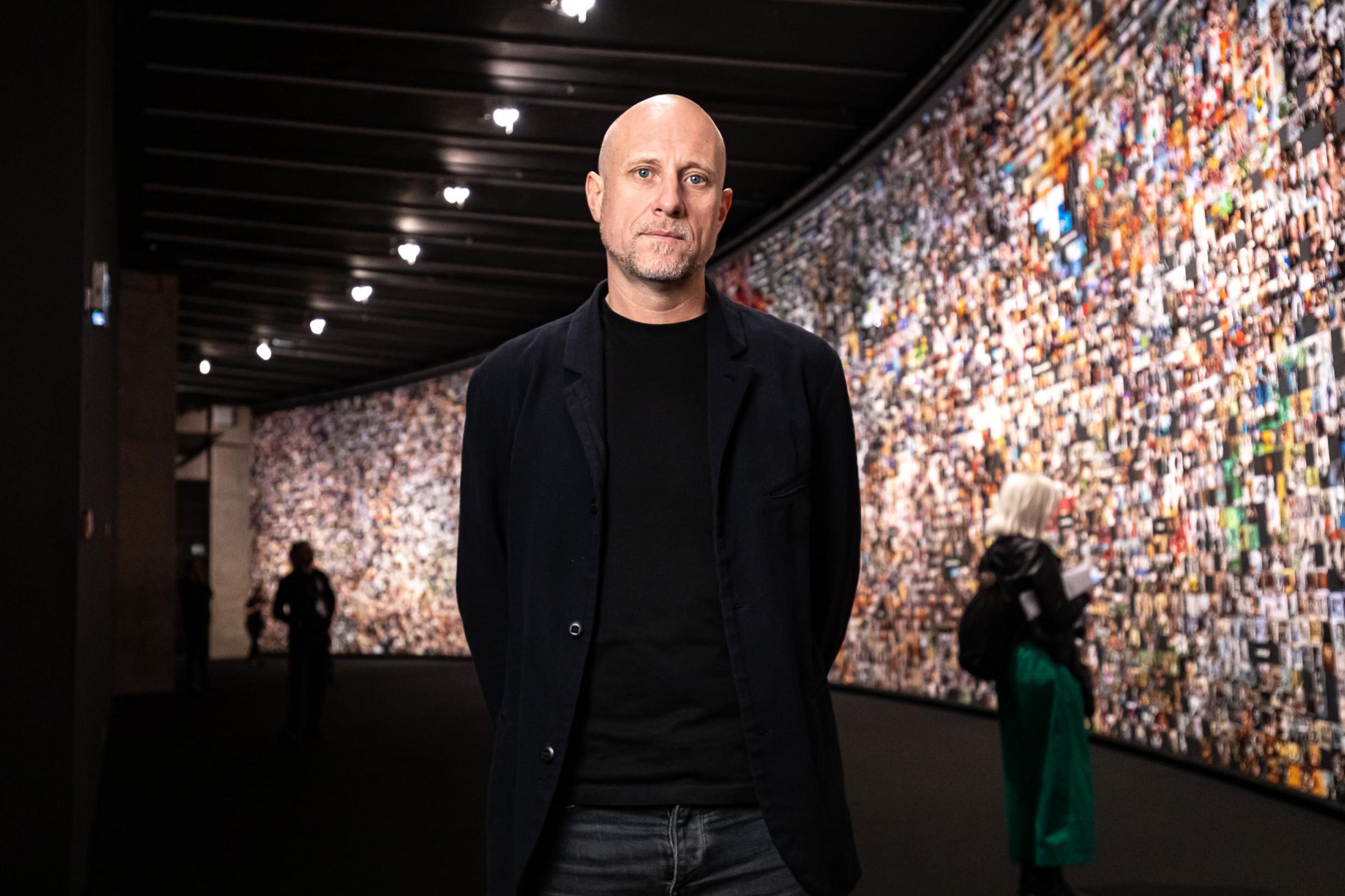
A classic example of this lies with a now ancient dataset known as Wordnet, which was put together in the late 1980s by George Armitage Miller – a progenitor of cognitive psychology. Miller was obsessed with words and wanted to explore the links between them, so he set up a dataset as a catalogue. Unfortunately, these words included an abundance of racist and misogynistic slurs, which went unnoticed by many programmers using Wordnet, and led to an apology from MIT after one of its datasets, which taught AI how to recognise people and objects in images, was found to be assigning racist and misogynistic labels.
There is a mounting pushback against the potential dangers of the dataset, including from an unlikely quarter – the art world. The MIT apology and eventual pulling of its problematic dataset was actually the result of a project by artist Trevor Paglen, who turned the dataset on its head in a piece called ImageNet Roulette. This uses “AI to identify any faces, then label them with one of the 2,833 subcategories of people that exist within ImageNet’s taxonomy”.
We need to make sure the politics of the dataset is brought under adequate scrutiny and to ensure AI does not inherit human psychopathy
According to API documentation, ImageNet is based on Wordnet 3.0, and it was this same “ImageNet taxonomy” that called Guardian tech reporter Julia Carrie Wong a “gook, slant eye”. ImageNet Roulette exposed the problem of biased datasets so plainly that it forced consequential change at a pivotal time in technology’s development. As Paglen puts it: “The rules and structure of the fast-developing computer vision are currently up for debate, and now is the time to engage.”
Another artist working to undermine the dataset is Jake Elwes; he’s endeavouring to turn it queer. Elwes sculpts his own datasets representative of queerness – by adding drag kings and queens to the set, he seeks to smother the AI in a thick layer of make-up – a project he calls Zizi.
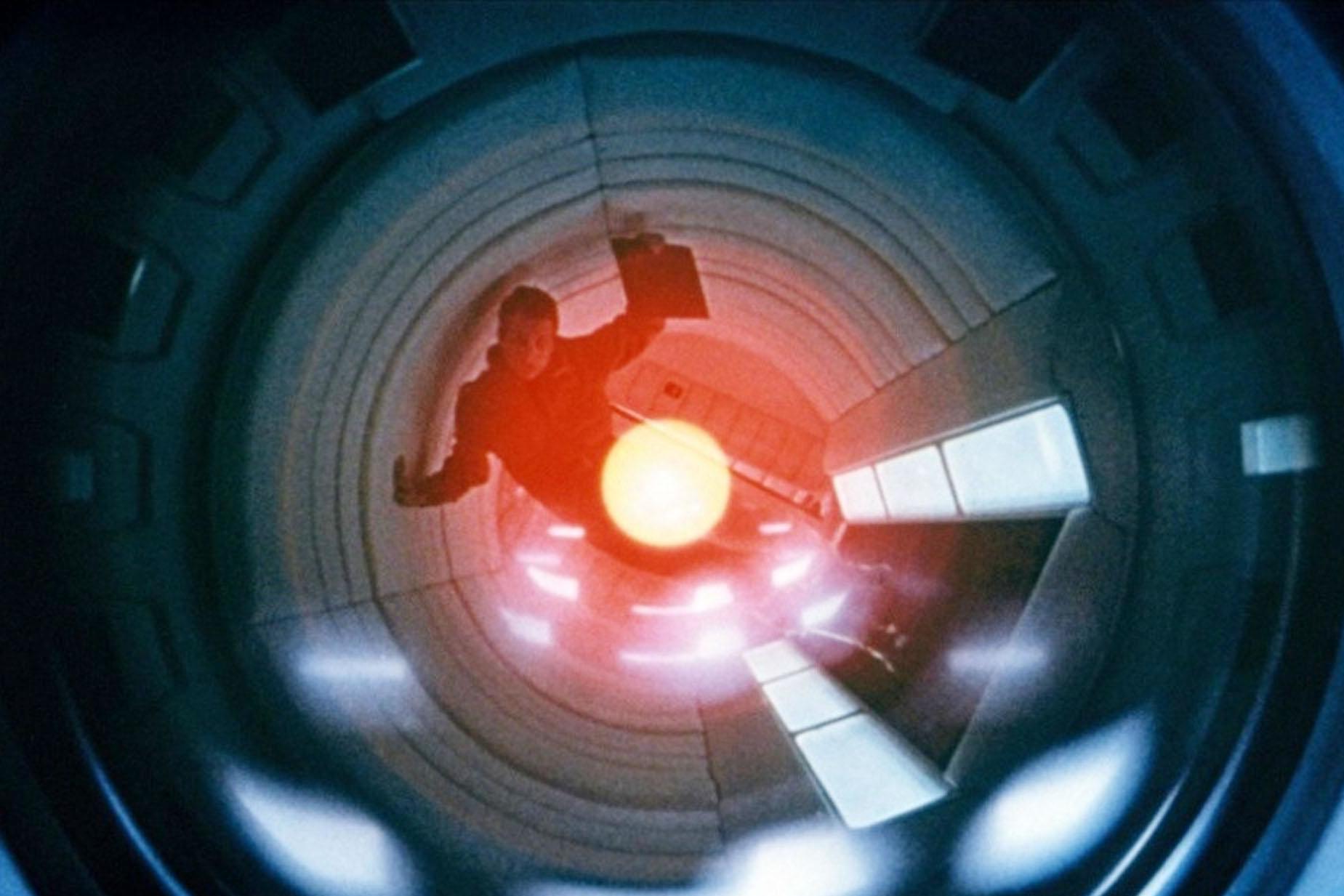
“Part of the political act,” Jake told me on a Zoom call from his north London home, “was the fact that I took a standardised dataset and did something else with it. I’m interested in the biases which exist in datasets which are being used to train systems by governments and big companies.”
Elwes then went on to give a description of ImageNet, which he sees as a deeply flawed system. “It’s put together with hundreds of hours of human labour, being paid minimum wage and contains such extreme words ... This is an algorithm that’s going to enforce the way we classify the world and if it contains a load of subjective terms like slut, drug addict, creep and loser then we’re in trouble. AI often has this mystification about it where it’s this autonomous thing. What’s much scarier is the fact that it’s actually a reflection of our own political views and biases.”
How we train our AI is a bit like how the assassins are trained in Parallax View (1973). We sit our systems down like Joe Frady in front of a slide show initiation, parading what we pretend is the full scope of human classification in front of them. Eventually classifications are lost from their original signifiers and, just like in the film, things have the potential of getting very dark, very quickly once subjective reality is – wittingly or unwittingly – muddled around with. We need to take every opportunity to make sure the politics of the dataset is brought under adequate scrutiny and to ensure AI does not inherit human psychopathy.
Join our commenting forum
Join thought-provoking conversations, follow other Independent readers and see their replies
Comments