Did the Office for National Statistics really produce ‘false data’ on coronavirus infections?
Some have suggested that dramatic data revisions on new coronavirus cases by the official statistics body indicate that the UK might have gone into lockdown in November unnecessarily. Do such claims have any substance? Ben Chu investigates
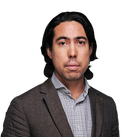

ITV’s political editor, Robert Peston, this week pointed to some spreadsheets produced by the Office for National Statistics which, he said, raised troubling questions about government policy during the pandemic, including whether the second lockdown was necessary or not to get infections under control.
The headline of his piece on the ITV website referred to “false data”.
The Daily Telegraph took up the theme on Wednesday with a story, based on the same spreadsheets, headlined with “Pre-lockdown spike did not exist, data shows”.
So what exactly is this “false data”? And does it really indicate that the UK went into lockdown in November unnecessarily? To begin we need to look at the source of the spreadsheets, which is the ONS’s Coronavirus Infection Survey.
Since this survey commenced in May, this has become one of the key official measures of how fast the virus has been spreading in the UK. It is a weekly study, covering tens of thousands of households across the UK, which have been chosen at random to participate.
Participating individuals are asked to give a sample, which is then tested to determine whether or not they are infected with coronavirus. ONS statisticians then extrapolate out from the results from the participating individuals to estimate the prevalence of the disease nationally.
This is similar to the way that opinion pollsters extrapolate from the results of their surveys of a sample of people, perhaps a thousand, to determine what the country overall is thinking on any given issue.
Such extrapolation methods might seem to be of questionable reliability – why would the view of a 1,000 random people reflect what a whole nation thinks? – but it’s well established in statistics that the larger the sample the more representative it will be, especially if the sample’s results are weighted and adjusted to reflect what we already know about the composition of the wider population.
And the ONS sample is sufficiently large and its geographical coverage sufficiently broad that statistical experts think it can be used to paint a reasonably reliable picture of what’s going on nationally (although there will always be some uncertainty about the precision of its estimates).
That’s all straightforward enough and this allows the ONS to produce an official weekly estimate of the number of people in the country infected with the coronavirus, and how that number has changed since the previous week.
Yet, separate from that exercise, the ONS also has a statistical model, which uses the weekly raw data from the survey to estimate the number of daily new cases of the virus.
And it is this model which has been the source of the concerns that have been raised.
The estimates produced by the model and published by the ONS on 26 November suggested around 45,700 new daily cases of coronavirus on 25 October in England, or around 8 per 10,000 people.
But the estimates produced by the model and published by the ONS on 4 December at 12.50pm suggests only 26,100 new daily cases on 25 October, or 5 per 10,000, which is clearly a rather significant difference.
Moreover, the most recent modelled results suggests the number of new daily cases was not rising in October, as previously shown, but rather flat.
This is the reason why The Telegraph article suggests there was no pre-lockdown spike.
So what’s going on here?
The ONS, for its part, has rejected this interpretation of its data, insisting people should distinguish between its official unmodelled estimates of incidence and the results thrown up by its statistical model.
The official estimates still point to a spike in cases in October, with a peak of 51,900 per day between 17 and 23 October.
And most experts agree that this is the correct reading of the data and most likely the true picture, pointing out that other surveys and studies also show sharply rising daily new cases through October.
“The ONS raw data is in agreement with Imperial College London's React study, the Covid symptom tracker and the government’s Covid dashboard which all suggest that infections were rising rapidly,” says Kit Yates, a mathematical biologist at the University of Bath.
So how to explain the fact that the ONS model shows something different?
The answer is that the model is set up with certain statistical assumptions to process often patchy raw data to produce an estimate of trends.
The ONS says that, as new, more up-to-date, data on infections (perhaps from swabs that come in later than others) is fed into the model, the results change, including the picture of what has been happening in the recent past.
Analysts say that’s fair enough in principle.
“Real time numbers are not as reliable as we would like and so it’s inevitable that there will be subsequent revisions,” says Flavio Toxvaerd, an economist specialising in economic epidemiology at Cambridge University.
We need to take ONS modelling, certainly their most recent estimates, with a pinch of salt
“This is completely commonplace. Once more data becomes available, you go back and set the record straight. The process of turning this information about the past into a forecast of where we’ll be going is what the models are for. And these are not perfect.”
The mathematician Sarah Rasmussen suspects one of the reasons the ONS model’s outputs have been volatile is that it has been rather confounded by new, incoming, data on infections among primary and secondary school children.
“Data trends from children have been qualitatively different to those from adults, and the model seems to have had a harder time coping with them,” she says.
Thomas House, a mathematical epidemiologist from the University of Manchester, says revisions are the price that must be paid for timely estimates.
“The size of the revision can be much bigger if there is more noise in the system, as there always will be for real data,” he says. “It doesn’t mean that any model involved was wrong, and simply reflects the finite nature of the sample.”
Yet, some experts feel that the results of the ONS’s model has been overly sensitive to new data, and that it should have been better calibrated so as not to produce such wild swings and, frankly, misleading estimates of recent trends and levels.
There may also be some lessons for the ONS when it comes to presenting their modelling data to journalists and the broader public.
The official unmodelled estimates and the results of the modelling data have been shown side by side on media releases by the ONS without a clear and upfront acknowledgement of the sometimes very different pictures they show.
More careful presentation might prevent the kind of misunderstandings we have seen this week.
“Generally, the ONS has been fantastic throughout the pandemic,” says Kit Yates.
“We just need to take their modelling, certainly their most recent estimates, with a pinch of salt.”
Join our commenting forum
Join thought-provoking conversations, follow other Independent readers and see their replies
Comments